In this article
Risk assessment is a primary management tool, and it plays a crucial role in various fields, providing valuable insights and helping organisations and government administrations to make informed decisions based upon the likelihood and impact of each risk. Although no individual or organisation, or even nation, can predict all specific risks, or what risks or vulnerabilities the future may hold, they can, and should, make preparations for any possibilities, probabilities and uncertainties. These preparations can be based on what they do already know, and what they can possibly predict for the future.
The COVID-19 pandemic has been the most significant risk to appear in the UK in recent times. The impact of COVID-19 affected all aspects of life, not only in the UK but also across the world. Risk assessments had identified a pandemic as a serious potential risk to the UK; however, it has been questioned in the subsequent inquiry whether the risk assessment took into account a broad enough range of possible scenarios in order to put in place effective plans to mitigate the impact of this risk.
Highly advanced cyber threats are now emerging as a pandemic that impacts and disrupts nations, government administrations, organisations and individuals, not only in the UK but also across the world, because of modern business practices such as globalisation.
Every day in the news we hear about issues that pose risks to nations, government administrations, organisations and individuals such as, but not limited to:
- Environmental issues such as global warming, adverse weather, natural disasters, air quality etc.
- Terrorist attacks
- International conflicts
- Strikes
- Inflation
- Diseases, pandemics, ageing populations
- Employment levels
- Skills shortages
- Crime, cybercrime, fraud
- New and emerging technologies
- Resource shortages
The world that we live in has changed rapidly and continues to do so. Technology innovation has brought new opportunities for connectivity, monitoring and awareness, and has changed and continues to change the way that we communicate and socially behave. Continuous advancements in technical knowledge and technology are improving our production processes, working practices and workplace locations, products and services, as well as our environment, healthcare and education.
However, all this fluctuating innovation is creating a complex landscape for risk assessment and risk management that requires a proactive rather than passive approach to identifying, assessing, mitigating and counteracting risks.
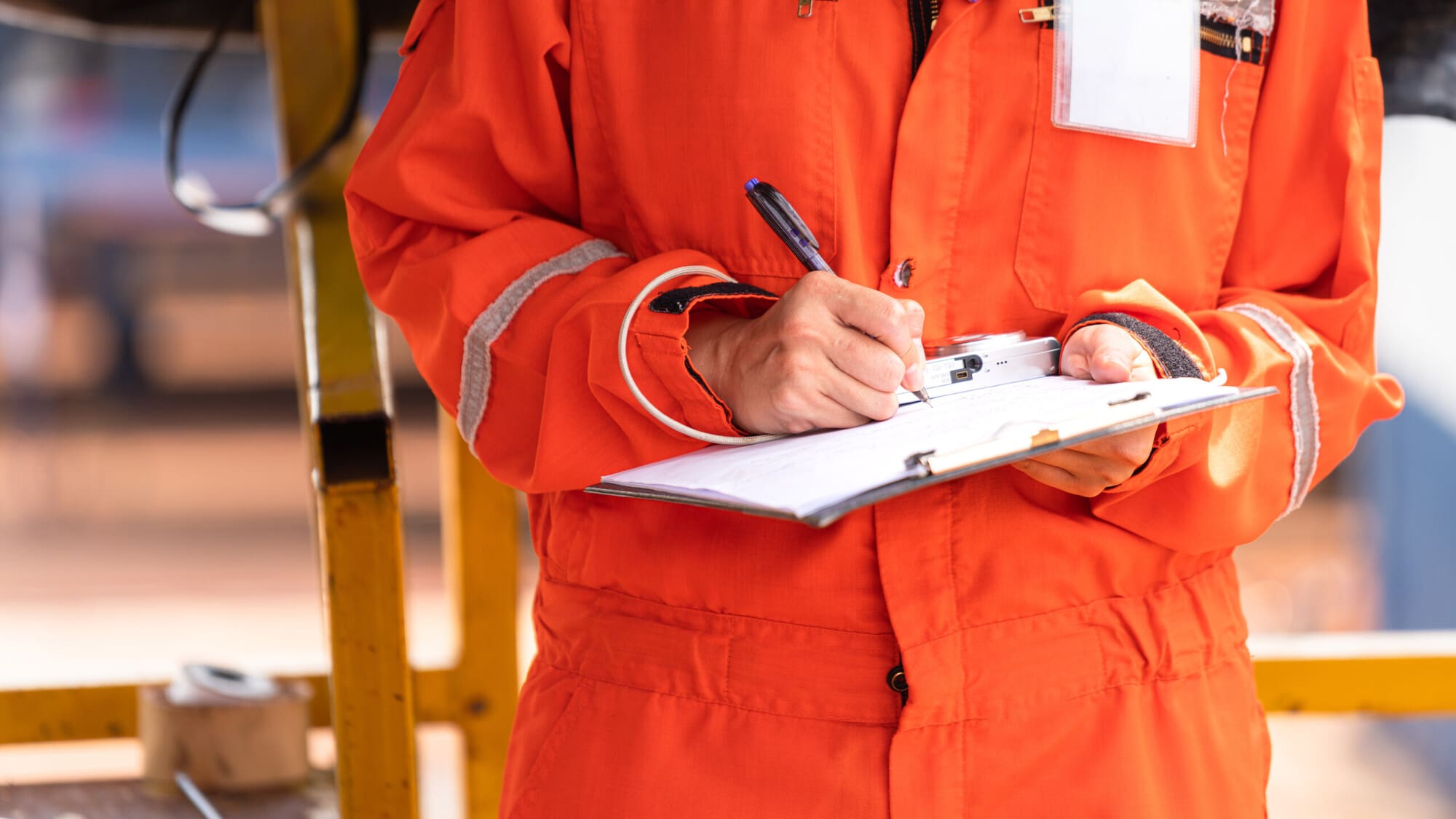
The evolution of risk assessment
Many academics have found examples of risk assessment dating back to ancient Athens, some two and a half thousand years ago, but it is probably much older, as making successful assessments of risks has been key to the survival of the human race over millennia. Humans have been using risk assessment to determine the dangers posed by the environment and their activities such as whether the risks posed by hunting for food outweighed the opportunities to be nourished.
Risk assessment and risk management has existed in the insurance industry for centuries, used when calculating premiums based on the probability of something occurring. So, risk assessment and management is a mature discipline that has evolved and expanded into many areas; although to a large extent, these ideas and principles still form the basis for the discipline today.
Risk assessment and management is primarily concerned with safety, whether that is the safety of, for example, people, environment, assets, data etc. And it was safety, more specifically Health and Safety at Work, that first saw risk assessment and management becoming a statutory requirement for organisations in the UK with the passing of the Health and Safety at Work etc. Act 1974 (HSWA).
Traditionally risk assessment methods have often relied on manual processes following the Health and Safety Executive’s (HSE) five-step process:
- Identify hazards
- Assess the risks
- Control the risks
- Record your findings
- Review the controls
Whilst the basic process remains the same, the use of risk assessment and management practices and tools has broadened, adapted and developed to incorporate many other safety issues facing organisations and government administrations, with risk assessment and management being factored into business planning, performance management, audit and assurance, business continuity management and project management to help combat modern challenges.
Risk assessment requires making a judgement on risk severity.
Risk severity = probability of risk materialising x impact of risk on, for example but not limited to, business activities, finance, assets, people, and other stakeholder interests.
Probability is usually categorised by levels and may be understood as:
- Low (Level 1) – a reasonably informed person would think it very unlikely this risk would materialise in the foreseeable future
- Medium (Level 2) – a reasonably informed person would think there is a significant possibility this risk would materialise in the foreseeable future
- High (Level 3) – a reasonably informed person would think there is a very significant or even likely possibility the risk would materialise in the foreseeable future
Impact is also usually categorised by levels and may be understood as:
- Low (Level 1) – any impact is minimal having regard to the importance of interests affected, impairment of function and duration. Typically, the impact is isolated and short-lived.
- Medium (Level 2) – any impact is significant having regard to the importance of interests affected, impairment of function and duration. Typically, the impact is limited to one function or group, but there is a material operational impact and the effects may continue.
- High (Level 3) – any impact is severe having regard to the importance of interests affected, impairment of function and duration. Typically, the impact impairs a critical function and/or has a systemic impact and the effects may be long-lasting or permanent.
Every organisation or government administration (entity) will face different types of risk. These can be:
Internal
These are risks over which the entity has some control, for example risks that can be managed through internal controls and, where necessary, additional mitigating actions. This often involves traditional risk management, such as risk registers, controls and assurance. Examples of internal risk might include, but are not limited to:
- Fraud
- Health and safety
- Capacity and capability
- Data security
- Delivery partners
External
This focuses on external risks and then considers how to make the entity more resilient to such events, in part because of difficulties in assessing likelihood. Consider the impact those external events could have on infrastructure, finance, people, operations, reputation etc. This forms a business continuity plan. Examples of external risk might include, but are not limited to:
- Economic downturn
- Terrorist attack
- Extreme weather
- Cyber-attacks
- Global pandemic
Strategic
This third element concerns the entity’s raison d’être and key objectives (such as the entity’s enduring purpose and the objectives set out in the Business Plan), identifying the principal risks to the achievement of those within a set timeframe. Risks in this area would be accompanied by regularly monitoring and adjusting interventions, as necessary. Forward-looking charts are often helpful here. Examples of strategic risk might include, but are not limited to:
- Immediate impact risks to the entity’s ability to continue operating, for example data governance and cybersecurity, business conduct, ethics and reputation
- Slow-burning risks that grow and eventually prevent delivery of objectives, for example key personnel turnover or leadership capability, political, economic and market exposure
Those arising from major projects – Major projects form such a critical part of the plans for many entities. These risks will be specific to the major project in question, and could involve but are not limited to:
- Fluid requirements
- Slippage in delivery timeframes
- Failure to deliver
- Contract disputes
- Finance
Assessing and managing risk is an ongoing process that has traditionally been triggered when changes affect the entity; however, this is being exceeded by a dynamic risk assessment which is a proactive, real-time risk management process designed to assess and respond to risks that can evolve rapidly or unexpectedly.
Nowadays, risk assessments need to be able to adapt to changes as they occur, making them particularly apt for high-risk and unpredictable environments. A dynamic risk assessment is often a more advanced approach to risk management that recognises the fluidity of risk factors, particularly in high-pressure or volatile environments.
All risk assessments should be regularly reviewed to verify if the control measures and level of evaluated risk are appropriate or require amendment.
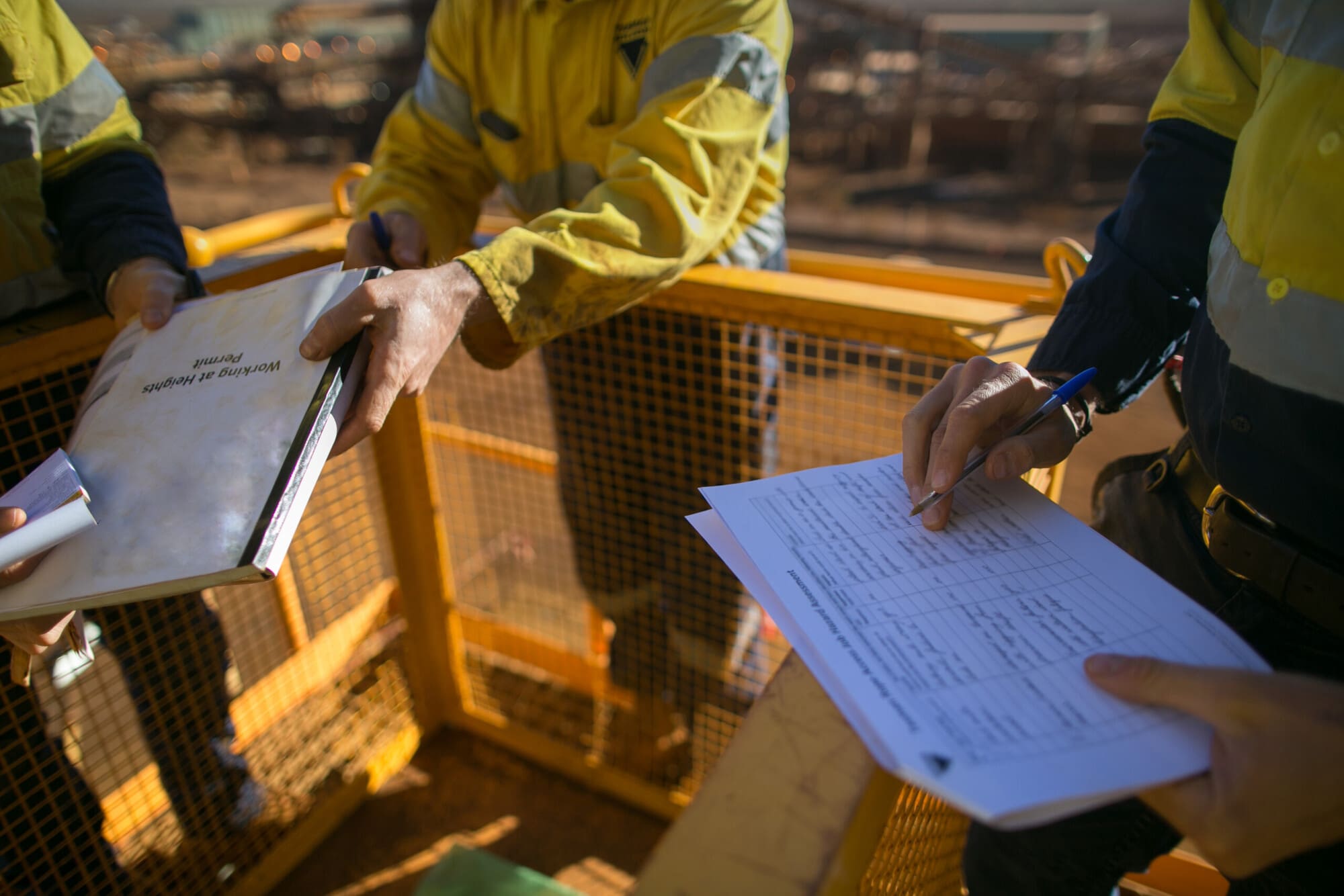
Data analytics and artificial intelligence (AI)
The innovation of artificial intelligence (AI) and data analytics is revolutionising our world and is beginning to impact every aspect of our personal and professional lives, and also impacting organisations and government.
Risk assessment and management analysis is largely about understanding the different types of risk lying before organisations and formulating the most appropriate methods to deal with them. To do this, data needs to be analysed; however, the sheer volume of data generated by most organisations daily has outstripped the capacity of human analysts to process and analyse. This data accumulation often includes network traffic logs, system logs, user behaviour data, and more. Human analysts can no longer sift through this data efficiently to identify anomalies and potential risks. AI, and particularly machine learning (ML) algorithms, has emerged as a potent tool to address these challenges.
AI-powered risk assessment systems excel at identifying patterns and anomalies within vast data sets. By analysing historical data and learning from it, AI algorithms can detect subtle deviations from the norm that might indicate a potential risk and enable organisations to identify potential risks before they escalate into full-blown issues.
Financial institutions, for example, are using AI to expedite and automate credit decisions by reducing manual data entry errors and risk. Instead of the rule-based decision-making of traditional credit scoring, AI can continually learn and adapt, improving accuracy and efficiency. AI is also a powerful tool for preventing fraud, using machine learning to identify trends and reducing false positives to detect transactions, behaviour, activity or content that looks suspicious or outside of the norm. There are a wide range of applications for AI in fraud and scam detection, for example bank transaction monitoring, spam message filtering, harmful content blocking, and for detecting malware.
AI systems and their capabilities present many opportunities, from expediting progress in pharmaceuticals to other applications right across the healthcare sector. Examples of AI which are currently being used to benefit people in health and care include analysing X-ray images, for example mammograms, to support radiologists in making assessments, and also an AI system might look to predict a patient’s length of stay at a hospital based on their previous admissions to hospital. Predictive AI can make accurate predictions and estimations about future events based on vast amounts of historical data. Researchers developed an AI tool using data from more than 200,000 A&E visits to a busy London teaching hospital, before and during COVID-19. The tool uses data on a person’s age, test results, how they arrived at A&E, and other factors to predict whether they will be admitted to hospital.
AI is helping NHS Trusts to allocate resources by identifying patients’ specific needs. For example, someone in their early 60s who doesn’t smoke and has low blood pressure would be seen as a “low risk” patient for surgery. They could have their operation sooner if they decide to have it done in a smaller hospital that might not have the resuscitation and intensive care areas that are found in bigger hospitals.
Without the AI-powered personalised risk assessment, a large number of patients currently have to wait longer for an operation appointment at larger hospitals. That is creating a huge demand for services in larger hospitals when many of those patients could be safely operated on in smaller hospitals. In larger hospitals the beds may be taken by other acutely ill patients so surgery is more likely to get cancelled. The AI risk assessment also allows patients at high risk of complications to decide whether they want the operation at all.
Predictive modelling and big data
Predictive modelling is a commonly used statistical technique to predict future behaviour. It works by analysing historical and current data and generating a model to help predict future outcomes. Risk prediction models use statistical analysis techniques and machine learning algorithms to find patterns in data sets that relate to different types of risks. However, the quality of data on which a predictive model is built and run will have an impact on the quality of the predictions it makes; high-quality data is the foundation of accurate risk prediction models. Relevant data sets need to be identified and pre-processed to address issues such as missing values, duplicates, inconsistencies and other data quality.
Predictive risk models can be used, for example, in healthcare for forecasting a range of healthcare and social care outcomes such as for predicting incidents like unplanned hospital admissions. In the business world, they enable data-based decisions to be optimised for particular risks and business opportunities as part of risk management initiatives. For example, credit risk models predict the risk of customer loan defaults, helping banks set credit limits.
As cybersecurity is a growing concern for all types of organisations and government administrations, risk prediction systems can detect anomalies and identify security threats before attacks occur. With predictive risk models continuously monitoring for business risks, organisations have the opportunity to respond faster to emerging threats and changing market conditions and this helps to build better business resilience.
Big data refers to the vast volume of organised and unstructured data generated and collected by organisations. Massive, complex data sets are rapidly generated and transmitted from a wide variety of sources such as emails, social media, videos and Internet of Things (IoT) sensors’ data. These can all constitute a big data stream, each with its own unique attributes.
IoT sensors are integrated into devices such as smartphones, doorbells, thermostats and even cars and refrigerators to capture important data that can be shared in real time with users. IoT sensors are also used in smart devices such as medical wearables, SMART metres and other monitoring tools used by individuals, organisations and government administrations. The data gathered by IoT sensors and sent to the cloud is analysed by software that can make sense of the information and then sent to users. This data is used to track trends and gather insights about everything from efficiency and energy use in homes, air temperature and traffic patterns, to a person’s vital signs, such as heart rate and oxygen levels, and a user’s health.
Big data is enormous. Whilst traditional data is measured in familiar sizes such as megabytes, gigabytes and terabytes, big data is stored in petabytes and zettabytes. To better understand the size of the data, one gigabyte is the equivalent of a seven-minute video in HD, whilst a single zettabyte is equal to 250 billion DVDs.
Although the large-scale nature of big data can be overwhelming, this amount of data provides users with a mass of information that can be used for creating insights for improving efficiency or predicting future outcomes. Big data analytics is used in nearly every industry to identify patterns and trends, answer questions, gain insights and tackle complex problems.
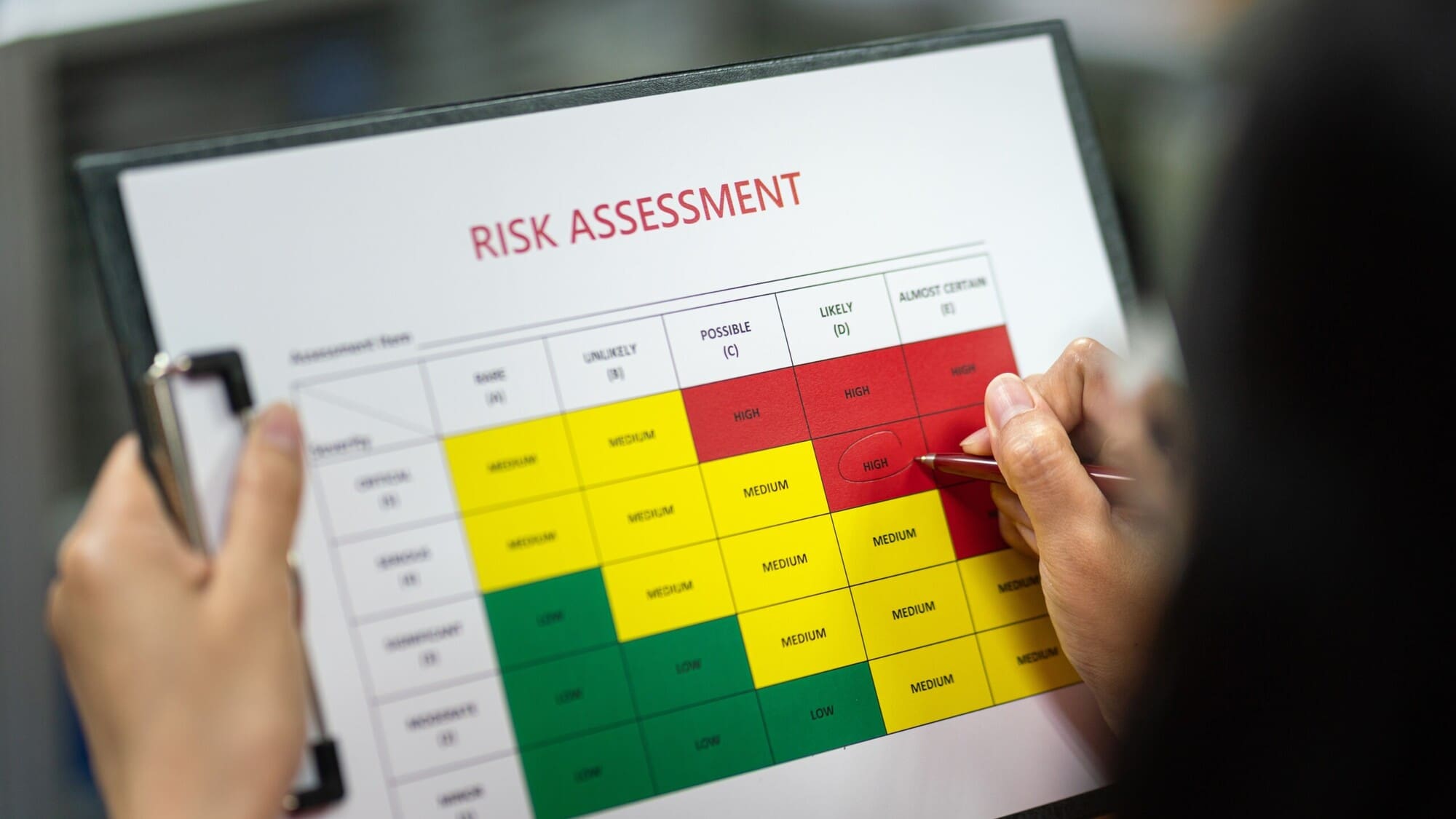
Behavioural analytics
Behavioural data is collected while a user engages with a website or digital application. Instead of capturing the specific information entered into form fields, behavioural analytics focuses on how the user interacts with the form. It analyses factors such as entry methods, whether they use autofill, typing, etc., how they edit, their fluency, timing, transition patterns and click rates. All of these can help to eliminate fraudulent applicants before the user even hits submit. Behavioural analytics connects a user’s behaviour to their intention, not their identity. Behavioural analytics does not require user profiles or personal data. It uses independent session engagement to distinguish between genuine and risky behaviour without storing personal data. It helps to detect genuine behaviour from risky behaviour based on independent session engagement.
In 2022, more than £1.2 billion was stolen from UK consumers through fraud, with nearly 80 per cent of APP (authorised push payment) fraud cases starting online. Behavioural data analysis prevents fraud in real time as it identifies devices with evasive behaviours, risky attributes or a history of fraud the moment they connect with a digital platform.
Because behavioural analytics analyses user behaviour data, it can quickly detect and reject bot activity that may otherwise have gone undetected. A bot is an automated software application that performs repetitive tasks over a network. It follows specific instructions to imitate human behaviour but is faster and more accurate. Malware or malicious bots perform activities that create security risks for organisations. They can disrupt operations, create unfair disadvantages, send out unwanted emails, or attempt unauthorised access to sensitive data. Fraud bots use artificial intelligence to mimic human behaviour to perform frauds.
Behavioural data analysis is key in combating cybercrime. Cybercrime involves gaining unauthorised access, or causing damage, to computers, networks, data or other digital devices, or the information held on those devices. Examples of cybercrime include hacking or unauthorised access into online accounts, for example banking, email or social media accounts, denial of service attacks, or devices being infected by a virus or other malicious software including ransomware. It was estimated that approximately 2.39 million cases of cybercrimes affected UK businesses over the past 12 months and that around 90% of UK organisations have encountered a greater risk of exposure to cybersecurity threats due to the rise of digital use over the past two years.
Behavioural biometrics are also used in risk mitigation; it verifies a user is who they say they are based on how they interact with their device compared to their past performance. This data generates a baseline of normal behaviour for each user. Any deviations from the baseline can then later be flagged as suspicious and investigated further. Examples of behavioural biometrics include keystroke, signature and voice data. The General Data Protection Regulation (GDPR) classifies biometric data as sensitive personal information requiring explicit consent for its processing including for identity verification and fraud prevention.
In the healthcare sector, the Office for Health Improvement and Disparities (OHID) brings together behavioural data and analysis on mental health and wellbeing from across the health and care system, and produces resources to help improve services and outcomes. OHID has created mental health profiling tools and guidance to help commissioners and other health professionals understand the mental health needs and services in their local area.
Blockchain and risk transparency
Blockchain technology is an emerging technology field, and one discipline that could take advantage of this technology is risk assessment. Blockchain technology can help by creating a secure and decentralised system that can be used to manage risks, rather than using a centralised database or server. Decentralised data storage eliminates the chances of a single point of failure, along with reducing the risk of data loss or corruption. During risk assessments, information collected can be stored on the blockchain, making it more secure and less vulnerable to attack.
The distributed nature of blockchain technology means that multiple stakeholders can access and update the data, improving collaboration and ensuring that everyone is working from the same information. Every transaction that occurs on a blockchain is recorded and verified by the network of nodes which means that there is an unalterable audit trail. Risk assessments, potential risks and vulnerabilities can be recorded and made tamper-proof, enhancing transparency and introducing accountability. Every stakeholder can have the capability to review the audit log and auditors can rely on this information and the risk assessment process.
Once the analysis is completed, the risk assessment data can be safely stored and distributed using the distributed ledger architecture of blockchain that provides a decentralised platform. All the nodes within the network will have the same information, which means that even if one node is corrupted, it will be extremely difficult for hackers to challenge the integrity of this data. The data is stored in blocks, which in turn are records of multiple transactions. They could neither be modified nor be blocked once it becomes a part of the ledger, making it tamper-proof. This is a secure way of record-keeping with no single point of failure.
Blockchain technology offers a more secure and transparent way of conducting risk assessments, reducing the possibility of data breaches, cyber-attacks, and other security threats.
Environmental and climate risk assessment
Climate change means that extreme weather incidents are becoming more common and more severe. Climate projections show that over the coming decades we will face an increased risk of climate change impacts, including:
- Extreme rainfall, leading to more frequent and severe floods
- Heatwaves
- Drought
- Rise in sea levels and tidal surges
- Storms
- Wildfires
All of these could have an impact on businesses directly, in supply chains and for consumers and markets.
Ongoing advances in technology, particularly in data analytics, machine learning (ML) and high-resolution climate mapping, are proving significant in risk assessment. These innovations enable organisations and government administrations to develop more sophisticated climate risk models that can assess a wide range of climate scenarios, and the integration of big data analytics and artificial intelligence (AI)-driven insights can make the assessment of climate risk more precise.
For the insurance industry, climate risks impact the financial system through two main channels: physical risks and transition risks. Physical risks arise from weather and climate-related events, for example rising sea levels due to melting ice caps. Transition risks arise as society adjusts to a low-carbon economy, including the risk that investments may lose value as a result, leading to so-called stranded assets.
For the agriculture industry, weather hazards, including heat, cold, wetness and drought, affect the viability of agricultural land through its yields of crops and livestock, and productivity overall. Extreme events and changing climatic conditions can present both risks and opportunities for agriculture.
The UK Government is required by the UK Climate Change Act 2008 to assess the risks and opportunities from climate change to the UK every five years and respond to the risks via a National Adaptation Programme, covering England. The devolved administrations also publish their own adaptation programmes in response to the risk assessment. The UK Government also publishes a National Risk Register; this is the 2023 edition.
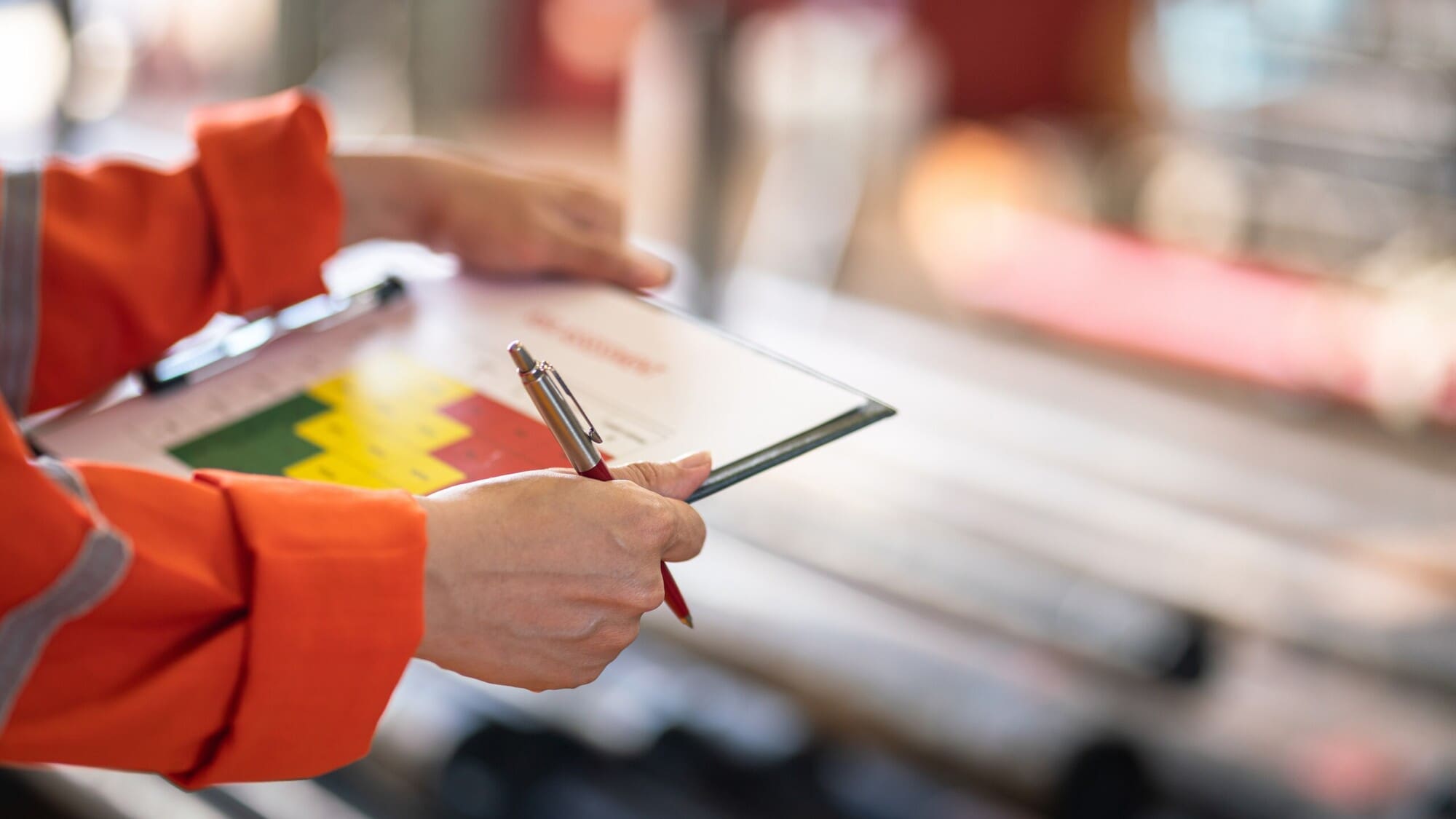
Ethical considerations and regulation
The use of data and automation has existed in some sectors for many years, but it is currently expanding rapidly due to an explosion in the volumes of available data, and the increasing sophistication and accessibility of machine learning algorithms. Data gives organisations a powerful weapon to see where bias is occurring and measure whether their efforts to combat it are effective. For example, if an organisation has hard data about differences in how it treats people, it can build insight into what is driving those differences, and seek to address them. However, there are numerous examples of where algorithms have entrenched or amplified historic biases, or have even created new forms of bias or unfairness, for example but not limited to:
- Representation Bias – this is where the input data does not accurately represent the real world, causing systematic errors in model predictions.
- Measurement Bias – this is where imperfect features and labels are used as proxies for the real variables of interest, resulting in incorrect measurements.
- Social Bias – this is where existing biases in human society are reflected in the available data, leading to replication and reinforcement of bias within the model.
- Evaluation Bias – this is where non-representative testing populations or inappropriate performance metrics are used to evaluate the model, leading to biased evaluations.
If biased information goes into an algorithm, the result will generally reproduce that bias. Using data that comes from a biased system will likely create an output shaped by the system’s bias.
It is important to recognise that biases can arise from both humans and machines within the socio-technical systems where algorithms operate. Addressing biases is crucial to prevent discrimination and unfair outcomes. Strategies such as diverse data collection and ongoing monitoring can mitigate biases and promote fairness in machine learning systems.
AI and ML can cause legal, ethical and fairness-related harms by unfairly allocating opportunities, resources or information, providing unequal service quality, reinforcing stereotypes, and under- or overrepresenting groups. Algorithmic bias qualifies as unlawful discrimination if it harms a protected group, falls within the scope of anti-discrimination law, such as the Equality Act 2010, and results in differential treatment or disproportionate disadvantage. It can also qualify for breaches of the Data Protection Act 1998 and 2018, and UK GDPR if, for example, data subjects are not made aware of the uses that their data may be subject to or are not asked for permission to process their data.
One of the key ways to mitigate the risks associated with AI is through regulation. Governments and other organisations need to work together to develop ethical frameworks and standards for the development and use of AI. This includes addressing the issues of bias and discrimination, as well as ensuring that AI is used in a transparent and accountable way. The UK Government has published an Ethics, Transparency and Accountability Framework for Automated Decision-Making; however, this is not currently enshrined in law. The Department for Science, Innovation & Technology has also published a white paper entitled ‘A pro-innovation approach to AI regulation’.
Conclusion
Risk assessment is developing and evolving at speed to keep pace with the fluctuating and dynamic nature of risks. The banking industry, which relies heavily on the use of data, is increasingly starting to adopt artificial Intelligence (AI) and machine learning (ML) techniques, and has started to leverage their powerful capabilities.
Across other industries and sectors, artificial intelligence is being increasingly recognised for its potential to significantly transform the day-to-day activities of an organisation or government administration. In risk management, AI and ML have become synonymous with improving efficiency and productivity while reducing costs. This has been possible due to the technologies’ ability to handle and analyse large volumes of unstructured data at faster speeds with considerably lower degrees of human intervention. The possibilities and promise for future innovations in risk assessment and management are exciting.