In this article
The concern about the rise in financial crime continues to grow as digital payments expand. Criminals are increasingly exploiting digital payment channels for money laundering and fraud, requiring stronger security measures to safeguard against these risks.
The UK is an international financial centre, which processes trillions of pounds of transactions from across the world every year. Together with the presence of a highly developed professional services industry, this significantly increases the vulnerability of the UK’s financial system to exploitation by those engaged in laundering the illicit funds of both UK and international criminals.
Fraud accounted for an estimated 41% of all crime experienced by adults in England and Wales in the year ending September 2022, and the National Crime Agency (NCA) assesses it is a realistic possibility that over £100 billion pounds is laundered every year through the UK, or through UK corporate structures using high-end money laundering methods. High-end money laundering is the laundering of funds, wittingly or unwittingly, through the UK financial sector and related professional services such as, but not limited to, insurance, accountancy, solicitors, private equity, brokers etc. This type of money laundering is particularly relevant in major frauds and overseas corruption, where the raw material of the crime is electronic and cash is only used further down the laundering process to disguise audit trails or to extract profits.
The exploitation of new and established technologies often allows criminals to stay ahead of the authorities, hide their activities and find new means of laundering the proceeds of their crimes. As criminals expand their capabilities, law enforcement needs to be agile in its approach to identifying threats and maximising technological opportunities.
The fight against money laundering has historically involved the manual scrutiny of financial transactions and customer activities, and the traditional methods of anti-money laundering has included stringent record-keeping, transaction tracking and customer due diligence processes, carried out by compliance officers to detect suspicious behaviours.
As money laundering is developing increasingly sophisticated laundering techniques, anti-money laundering prevention and detection, primarily driven by artificial intelligence (AI), machine learning and data analytics, is being revolutionised to meet these new demands.
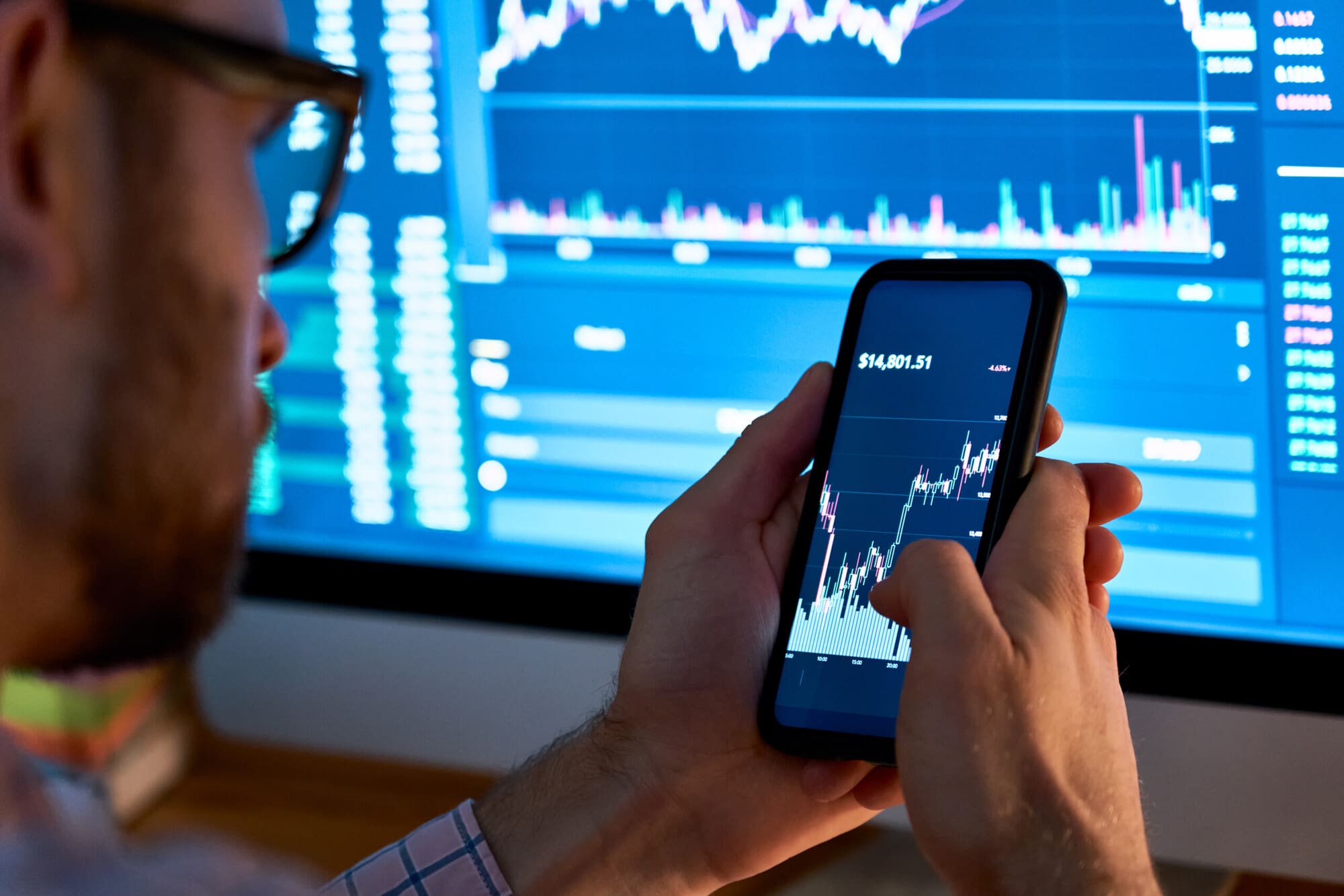
The Evolution of Money Laundering
Money laundering is an age-old practice, possibly dating back hundreds of years where illegally obtained money has needed to be hidden from view of the authorities and to appear to be legitimate when it comes back into view. Urban myth has it that the term money laundering itself was first coined when US authorities were investigating the dealings of criminals such as Al Capone. These criminal factions were cleaning the money gained from bootleg alcohol and protection rackets during the prohibition period through chains of laundromats set up to disguise the origin of the money. Laundromats are cash enterprises and the illicit cash was simply added to the laundromats’ revenue and re-introduced into the financial system as clean money, hence referring to the practice as money laundering, a term that continues to today. Interestingly though, Capone was not charged or convicted for money laundering, as at that time it was not considered a serious crime. His conviction was for tax evasion, a more serious offence in 1931.
Although prohibition ended in 1933, the practice of money laundering continued not only in the US but also around the world, and money laundering regulations first came into force in the UK in the 1930s.
In the 1980s money laundering was used by criminals largely for laundering the proceeds of drug trafficking, and the drug trade and money laundering had evolved into international crimes. An important development to help fight organised crime and money laundering took place in 1989 when the G7 States, including the UK, decided to set up a Financial Action Task Force (FATF) to combat the growing threat of money laundering.
By 2001, following the 9/11 attacks, the financing of terrorism became an additional concern for money laundering prevention. Legislation was passed and subsequently amended to take into account the ever-changing landscape of serious organised crime and money laundering. The UK anti-money laundering regime requirements are set out in the Proceeds of Crime Act 2002 (POCA), as amended by the Serious Organised Crime and Police Act 2005 (SOCPA), the Money Laundering, Terrorist Financing and Transfer of Funds (Information on the Payer) Regulations 2017 (MLR 2017) and the Terrorism Act 2000 (TA 2000) as amended by the Anti-Terrorism, Crime and Security Act 2001 (ATCSA 2001), and the Terrorism Act 2006 (TA 2006).
Over the almost 100 years since the first money laundering regulations in the UK, there have been numerous revisions made to these laws to take into account developments in money laundering techniques and technologies. The last 5 years have seen the introduction of supplementary guidance and regulations that take into account the more sophisticated money laundering methods that are being applied:
- Criminal Finances Act 2017
- The Money Laundering and Terrorist Financing (Amendment) Regulations 2019
- Money Laundering and Transfer of Funds (Information) (Amendment) (EU Exit) Regulations 2019
- Anti-Money Laundering Guidance for the Accountancy Sector (AMLGAS)
- The Money Laundering and Terrorist Financing (Amendment) Regulations 2022
- The Economic Crime and Corporate Transparency Act 2023
From 1 April 2006 until 7 October 2013 the Serious Organised Crime Agency (SOCA), a UK national law enforcement agency, had responsibility for investigating and preventing serious organised crime including money laundering. Serious organised crime such as money laundering and terrorism-related offences is now investigated and prosecuted by the National Crime Agency (NCA), who work on an inter-agency basis both nationally and internationally. Within the NCA, the National Economic Crime Centre (NECC) has been created to deliver a step change in the UK’s response to, and impact on, economic crime.
For the first time, the NECC brings together law enforcement and justice agencies, government departments, regulatory bodies and the private sector such as the Home Office, GCHQ, HM Revenue and Customs (HMRC), and the Financial Conduct Authority (FCA), with a shared objective of driving down serious organised economic crime such a money laundering. Financial crime continues to be a focus for the Financial Conduct Authority (FCA), as according to its latest annual report, of its open cases, 162 were criminal and 138 were “dual track” (both criminal and regulatory) investigations.
As digital transactions have created new and sophisticated opportunities for money launderers to operate, national inter-agency and international cooperation and partnership, and the utilisation of ever-developing technology, are vital to combating digital money laundering and serious organised crime.
The Technological Arsenal Against Money Laundering
The UK is leveraging advanced analytics solutions to address emerging money laundering and terrorism financing risks and to strengthen anti-money laundering efforts. The United Kingdom Payment Market size is estimated at USD 490.77 billion in 2024. It has experienced significant growth and change in recent years, and is expected to reach USD 849.64 billion by 2029.
Almost nine out of 10 payments were made by consumers, and 57% of all payments were done using cards, with 66% of those being contactless transactions. This shows the increasing popularity and ease of modern payment methods. As digital payments continue to grow, so does the concern about the rise in financial crime related to these transactions. Criminals are increasingly exploiting digital payment channels for money laundering and fraud, necessitating stronger security measures to safeguard against these risks.
A steady increase in the use of cryptocurrencies and the creation of countless crypto-related businesses and exchange companies have been exploited by criminals laundering illicit funds.
Traditional anti-money laundering (AML) methods have been particularly labour intensive and have been costly, whereas new and emerging technologies have genuine potential to have a transformative impact on AML compliance, both in helping to prevent money laundering and in reducing the cost of compliance.
Emerging trends in anti-financial crime include more investment in and integration of artificial intelligence (AI). AI’s role in AML is multifaceted, offering enhanced detection capabilities, predictive analytics and dramatically improved efficiency in identifying illicit financial activities.
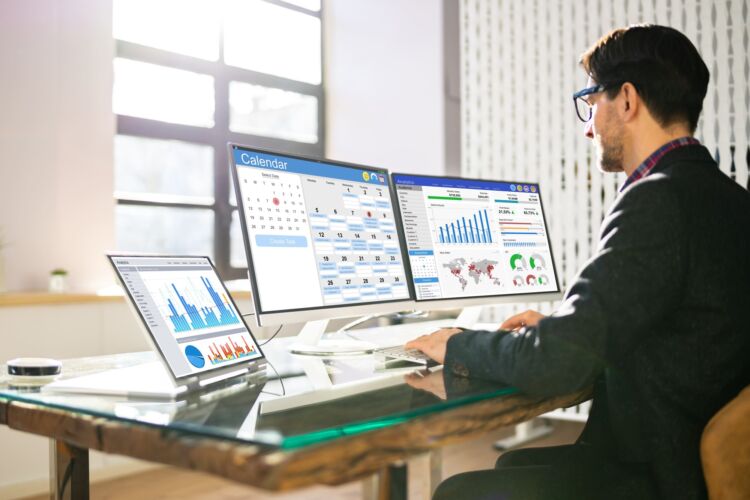
AI and automation enhance controls, productivity and risk mitigation. AI-driven tools help to identify unusual patterns in transactional data, helping to flag potentially suspicious activities. It also can be used to streamline AML processes, reducing the time and effort required to review transactions and ensure regulatory compliance. AI-powered systems can continuously monitor transactions, identifying unusual patterns and potential threats thereby increasing fraud detection capability.
Automation excels in optimising routine, rule-based tasks, something that AI also excels at. Tasks ranging from customer onboarding processes to transaction monitoring and anomaly detection can now be automated. The use of AML technologies for customer onboarding and maintenance is seen as part of a broader shift towards digitisation.
Recent enhancements in machine learning (ML) are helping banks to improve their anti-money-laundering (AML) programmes significantly, including, and most immediately, the transaction monitoring element of these programmes. Transaction monitoring refers to the monitoring of customer transactions including assessing historical/current customer information and interactions to provide a complete picture of customer activity. This can include transfers, deposits and withdrawals. With machine learning (ML), powerful algorithms are used to extract patterns that are historically associated with suspicious activity.
Whilst rule-based system designs may be the most straightforward way to translate concerns into an actionable system, they suffer from many downsides. For example, rule-based systems can be easily circumvented by money launderers who are familiar with the rules, or they do not consider the full range of money laundering indications because they are programmed to respond to specific conditions. The ML approach to automated pattern identification leads to more precise and effective screening, and removes the need to manually define rules to sift through data. ML can be an effective way to combat money laundering and ensure that financial institutions are compliant with regulations.
Blockchain technology possesses inherent characteristics that can potentially prevent money laundering. Every transaction done over blockchain leaves behind a permanent trail of records which is impossible to alter, making it easier for authorities to track the original source of the money. A public blockchain ledger can supervise, validate and record each transaction’s complete history. The public ledger’s readers and crypto miners get immediate notification of transactions as they occur. If all the transaction phases, including destination wallet, departure wallet, currency type and amount, are anyhow left unverified, the transaction gets immediately blocked.
Blockchain also enables money laundering risk analysis and reporting mechanisms. It allows overall system analysis rather than monitoring just entry and exit points. Integration of Smart Contracts in a Blockchain-based AML platform automates the process of fraud detection in the system. In-built algorithms keep a continuous check on every transaction, automatically generate an alert for a suspicious transaction and immediately block the transaction. The data recorded on a blockchain ledger cannot be altered or removed but is always transparent to all members of the network.
Data analysis plays a crucial role in the field of anti-money laundering (AML) by enabling financial institutions to effectively detect and prevent financial crimes. By leveraging big data analytics, AML professionals can uncover patterns, detect anomalies, and manage risks more efficiently. Data analysis in AML involves the systematic examination of transactional data, customer profiles and other relevant information to identify suspicious patterns or behaviours. Through the use of sophisticated algorithms and statistical models, vast amounts of data can be analysed in real time, enabling quick identification of potentially suspicious activities.
Data analysis allows financial institutions to identify complex patterns and anomalies that may indicate money laundering activities. It can reveal hidden relationships and networks involved in money laundering. Through entity resolution techniques and network analysis, financial institutions can identify connections between individuals, organisations and transactions. By analysing large volumes of data in real time, financial institutions can generate alerts for further investigation and intervention, leading to more effective detection of suspicious activities.
To effectively combat money laundering and meet regulatory compliance requirements, organisations are increasingly utilising advanced technologies to efficiently identify patterns, detect anomalies, and manage risks effectively in their anti-money laundering (AML) efforts. These techniques enable organisations to extract meaningful insights from complex datasets, improving their ability to detect suspicious activities and mitigate risks effectively.
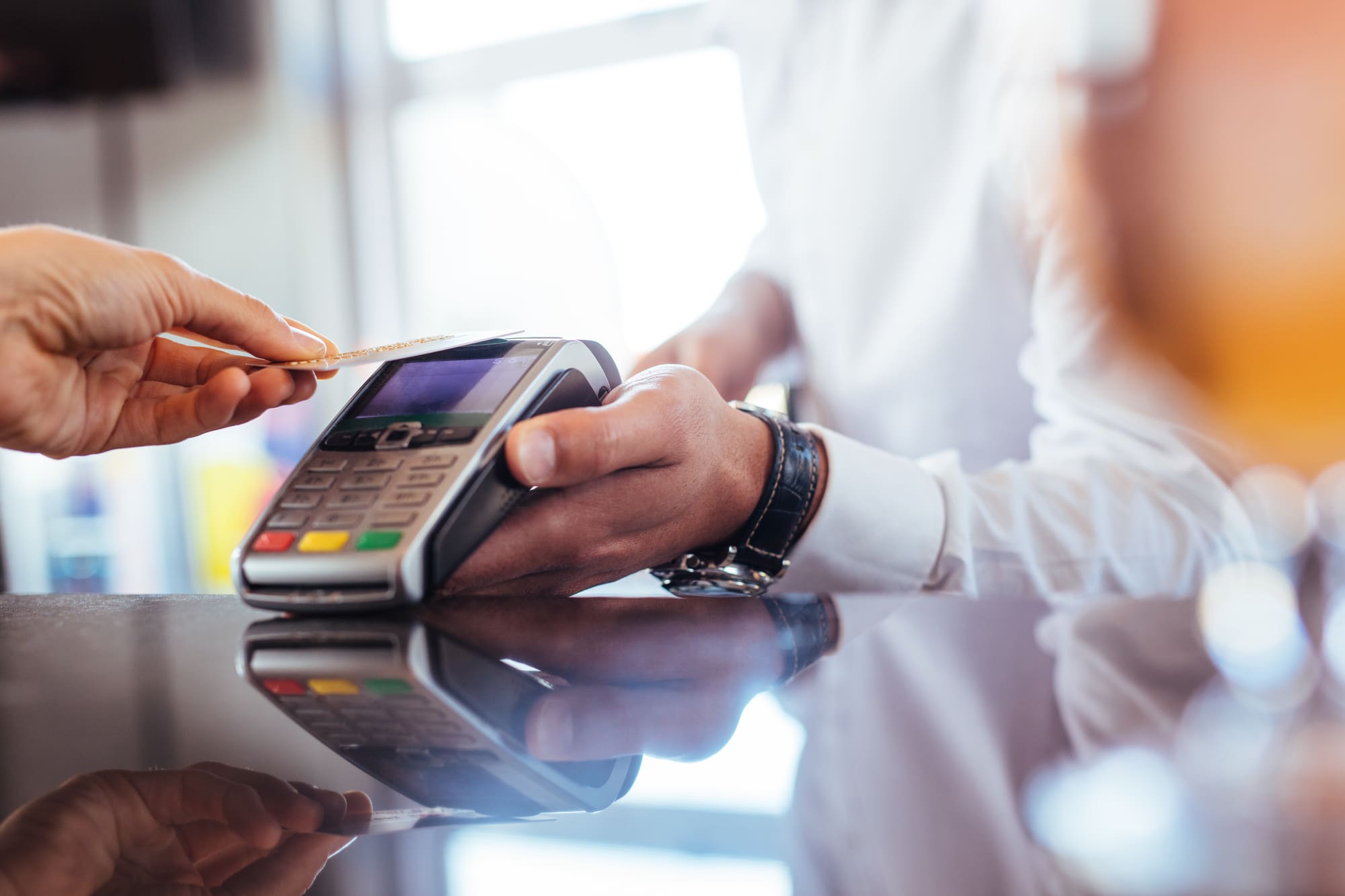
Real-Time Transaction Monitoring
Real-time transaction monitoring is a proactive approach used by financial institutions and businesses to scrutinise every transaction as it happens. By constantly analysing transactions as they occur, organisations can detect and respond to suspicious activities in real time, preventing potential losses and safeguarding the integrity of their operations.
When a transaction occurs, whether it is a credit card purchase, an online transfer, or any other financial activity, the transaction data is instantly captured and sent to the monitoring system. This system then applies a series of sophisticated algorithms to analyse the data in real time. These algorithms consider various factors, such as the transaction amount, the location of the transaction, the customer’s historical behaviour, and any patterns or trends that may be indicative of fraudulent activity. The system compares the current transaction against a vast database of known fraud patterns and uses machine learning techniques to identify new and emerging fraud patterns. Once the system detects a potentially fraudulent transaction, it triggers an alert to the organisation’s fraud detection team, who can then review the transaction in detail, gather additional information if necessary, and make an informed decision on whether to block the transaction or allow it to proceed. This entire process happens within seconds, ensuring that fraudulent activities are identified and addressed in real time.
A real-time transaction monitoring system tracks every piece of data associated with a transaction and transmits that information into risk rules. Using these rules, the system automatically flags or blocks suspicious actions such as:
- Sources of inbound and outbound funds that are unknown
- Transactions that exceed a certain threshold
- Transfers beyond a certain value, whether domestic or international
- Unusual account activity or transactions
- Significant cash deposits or withdrawals
Most of us have been the subject of transaction monitoring at one time or another. For example, if you have ever received a call from your financial institution requesting that you confirm specific transactions, it is because the financial institution has a good knowledge of what your regular financial activities are, and is alerted through real-time transaction monitoring to anything out of the ordinary or suspicious. If they notice unusual activity, for example large cash deposits or money transfers, they might contact you or, in some circumstances, report it to the authorities if suspicious activity is suspected.
More than 118 billion real-time transactions were made in 2021, according to ACI Worldwide and Global Data research, up from 70 billion in 2020. That number is expected to grow to more than 427 billion by 2026. The rise of real-time instant payments has opened new avenues for criminals to exploit. Instant or real-time payments are convenient and efficient, allowing a person to receive money in seconds. However, this leads to various new fraud scenarios, where authorised or unauthorised parties are either tricked into sending money, or they knowingly participate in fraudulent activities.
Real-time instant transactions have increased the need for real-time transaction monitoring, as knowing how to recognise a suspicious transaction could prevent fraudsters from laundering large sums of money. The essence of real-time transaction monitoring lies in the speed of the system’s response, which needs to be extremely fast, typically in milliseconds. With the rise of instant real-time payments, financial institutions often have only seconds to decide whether to accept or reject a payment. It is crucial that these checks are done in milliseconds, at a point when reversing the payment is still possible, thereby minimising the customer impact. Having real-time transaction monitoring in place provides confidence not only to customers but also to regulators and to financial partners.
Organisations such as PayPal and Visa use real-time transaction monitoring to proactively detect and prevent fraudulent activities, safeguarding their financial assets and maintaining trust with their customers. By actively monitoring transactions, these payment processors can identify potential fraud in real time and take immediate action to block suspicious transactions, safeguarding the financial interests of merchants, but also protecting consumers from unauthorised charges or fraudulent transactions. PayPal’s system analyses over 25 billion transactions per year, using advanced analytics and machine learning to identify and prevent fraudulent activity. Visa’s system analyses over 500 million transactions per day, using advanced analytics and machine learning to identify and prevent fraudulent activity. These real-world examples demonstrate the importance of real-time transaction monitoring in combating fraud.
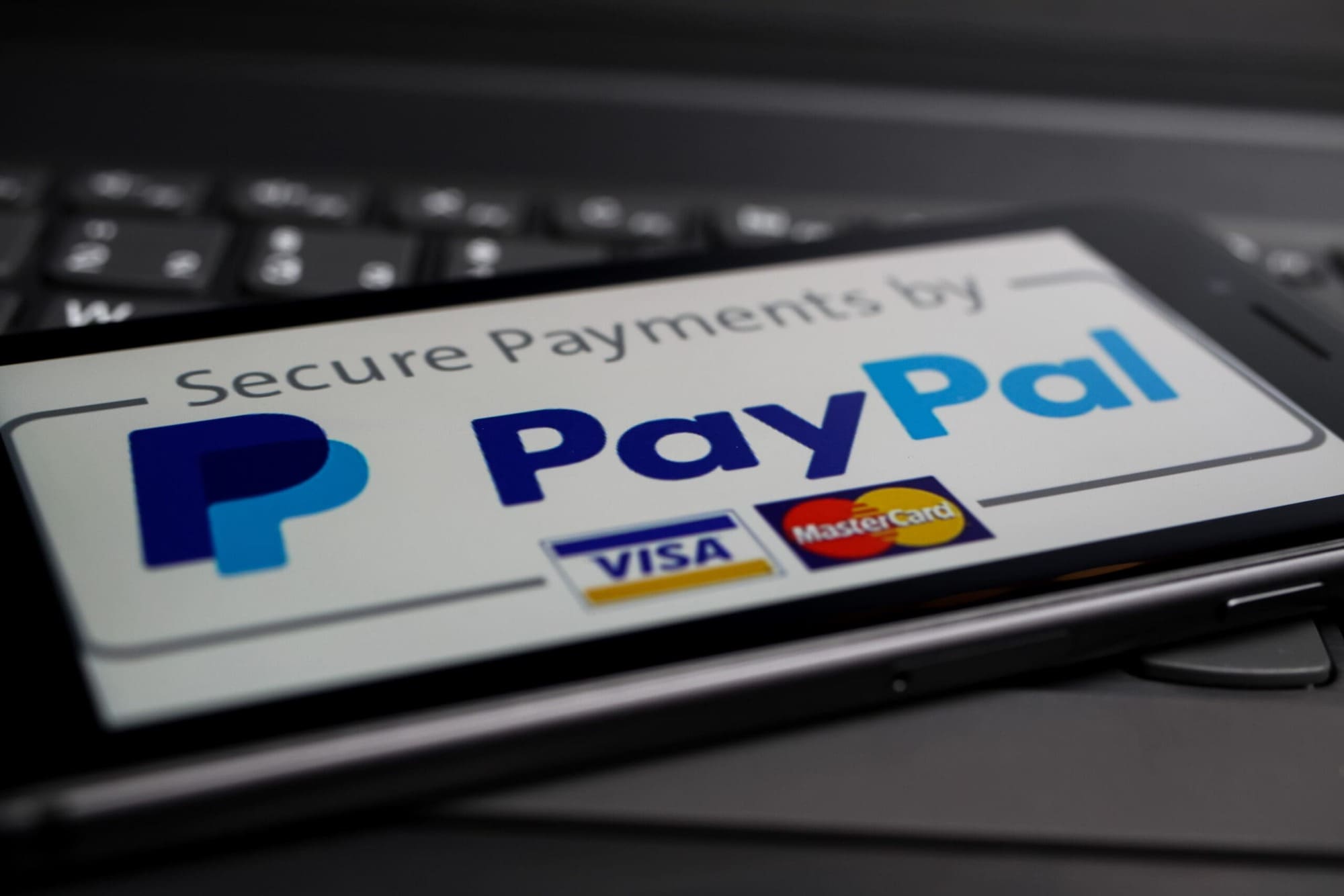
Blockchain and Cryptocurrency Tracking
The widespread use of cryptocurrencies for illicit financing has created huge challenges for law enforcement agencies who are working to trace funds and identify the criminals involved. The pseudo-anonymous nature of crypto transactions generates a considerable smokescreen for these transactions, especially when sophisticated tactics such as mixers are employed. However, advanced blockchain analytics techniques can help to illuminate crypto cross-chain transaction trails and unlock crucial investigative insights.
Blockchain creates a transparent and immutable ledger for transactions; however, it also has the potential to prevent crime. In blockchain technology, each transaction is time-stamped and linked to the previous one in a chain of blocks. Blockchain technology offers a high level of security due to its decentralised nature and cryptographic algorithms. This can prevent unauthorised access to sensitive data and prevent cyberattacks. This transparency can be leveraged to identify and track illegal activities such as illicit cryptocurrency transactions on the dark web.
The dark web is part of the internet that isn’t visible to search engines and requires the use of an anonymising browser called Tor to be accessed. The terms “deep web” and “dark web” are sometimes used interchangeably, but they are not the same. Deep web refers to anything on the internet that is not indexed by and, therefore, accessible via a search engine such as Google. The dark web is a subset of the deep web that is intentionally hidden, requiring a specific browser to access it. Some, but not all, of the dark web is being used for criminal purposes such as illicit cryptocurrency transactions.
Dark web users who utilise blockchain technology can benefit from the security features built into the blockchain, reducing the risk of data breaches and hacks. This added layer of security can discourage criminals from engaging in illegal activities. Some blockchain companies are actively collaborating with law enforcement agencies to combat criminal activities on the dark web using advanced blockchain analytics tools to trace transactions and identify potential criminals.
Blockchain analytics tools are a valuable resource for law enforcement agencies in investigating and tracking cybercrime activities. These tools can be used to monitor seized assets using blockchain data. Blockchain forensics provides transparency into an otherwise opaque ecosystem, arming agencies with intelligence to combat crypto-enabled crimes. Investigators can gain valuable crypto insights to fast-track organised crime, cybercrime, terror and money laundering investigations.
By using blockchain analytical tools, law enforcement agencies can trace the flow of cryptocurrency transactions, discover real-world identities of blockchain addresses and wallet holders, and scan crypto wallets for AML and sanctions compliance. Law enforcement agencies can also use blockchain analytics to track down crypto criminals by identifying suspicious addresses and providing actionable insights to assist in asset recovery efforts.
As blockchain tracking has grown more sophisticated, criminals have adopted anonymisation techniques to avoid detection such as transaction fracturing which seeks to avoid scrutiny by spreading amounts across many outputs and chains. However, advanced clustering algorithms such as pattern analysis, activity monitoring and timing correlations can often reconnect the dots and can accurately group related wallets and map fragmented activity trails.
From 1 September 2023, the Financial Action Task Force’s (FATF) Travel Rule requirements for crypto assets came into force in the UK. The Travel Rule is the new Part 7A of the Money Laundering, Terrorist Financing and Transfer of Funds (Information on the Payer) Regulations 2017 (MLR). It requires UK-based crypto asset businesses to collect, verify and share specific transaction information relating to transfers of crypto assets. It advances anti-money laundering and other financial crime efforts by requiring crypto asset businesses to detect suspicious transactions and carry out effective screening. UK-based crypto asset businesses must comply with the Travel Rule before permitting an inter-crypto asset business transfer and should verify the information it receives on the basis of a reliable source which is independent of the person whose identity is being verified.
However, the pseudo-anonymity of crypto assets is a foundational attraction of the technology. In many instances, this only requires the wallet address of the beneficiary and private cryptographic key of the originator and as such can make the Travel Rule an inherently difficult requirement for crypto asset businesses to fulfil. Combining emerging analytics with collaborative partnerships such as those formed through the global implementation of the Travel Rule, promises to overcome the use of cryptocurrencies for illegal financing and money laundering.
Future Possibilities and Ethical Considerations
Fraud prevention and anti-money laundering will continue to be complex as digital technology evolves. The advancement of technology has provided fraudsters with more sophisticated tools and techniques to exploit vulnerabilities in transactional systems. It is therefore crucial that organisations have robust fraud prevention strategies and innovative solutions such as real-time transaction monitoring, AI, blockchain, machine learning and data analysis which are essential to keep pace with, or rather to outpace, technologies being used and developed by fraudsters and criminals. However, increasing data privacy considerations may prevent the development of more efficient and cheaper options for analysing data.
On a global scale, there is no legal authority dealing with individual privacy. Instead, there are territorial privacy regulations that apply only to specific countries or regions. In the UK these are the Data Protection Act 1998 and the UKGDPR 2018. These laws and regulations make it legal to collect, use and store the personal information of legal persons. Even though the GDPR has been implemented and is in effect in the UK and in Europe, it has far-reaching effects. Its scope extends outside the UK and the EU, and businesses providing goods or services to UK or EU nationals for the purpose of monitoring their online behaviour must comply with it regardless of location.
There has, however, been a battle between privacy and transparency in the fight against financial crime. The 5th Money Laundering Directive in the EU introduced the requirement for open, freely accessible public Ultimate Beneficial Owners (UBOs) corporate registers across all member states. In the UK this directive is known as The Money Laundering and Terrorist Financing (Amendment) Regulations 2019. However, in the EU a recent European Court of Justice ruling debuted, which stated that the requirement of the 5th Money Laundering Directive for open public registers of UBOs is invalid and an affront to the right to privacy of the UBOs of companies. Since this ruling, some EU jurisdictions have restricted public access to corporate registries. This is not the case in the UK; beneficial ownership registers are kept by Companies House and failure to declare a registrable beneficial owner is a criminal offence.
This battle of privacy versus information sharing is also played out in private information-sharing initiatives. There is an increased desire in the financial industry to share information on suspicious activities, criminal entities or individuals. Several initiatives where data is transferred or monitored collectively have been established for both Know Your Customer (KYC) and transaction monitoring. Until the matter of protecting Personally Identifiable Information (PII) is resolved or there is a clear legal gateway to share information, genuine information-sharing initiatives will struggle to get off the ground, and many will fall at the first hurdle. This is a huge challenge for law enforcement and a bonus for hackers, scammers, money launderers and cyber-enabled fraudsters.
Fraud and money laundering are ever-changing and increasing threats to both individuals and organisations. As a result, a wide array of biometric verification and authentication methods have developed over time, and ensuring robust identity verification measures has become vital. Facial biometrics refers to the use of unique facial features to identify individuals. It involves capturing and analysing facial characteristics such as the distance between the eyes, the shape of the nose, and the contours of the face. This information is then used to verify or authenticate a person’s identity. Facial recognition technology is frequently used for security measures, entry management, and identity verification across different sectors.
Facial biometrics technology provides a potent arsenal for identifying suspects, monitoring individuals, and swiftly detecting fraudulent activities such as fraudulent transactions, unauthorised access, identity theft and money laundering. However, implementing facial biometric systems for identity verification can pose several challenges, ranging from technical limitations to privacy concerns and bias.
Human faces exhibit a wide range of diversity in terms of ethnicity, age, gender and facial features. Recognising and accurately identifying individuals across diverse demographic groups poses a significant challenge for facial biometric systems, which must account for this variability to avoid biases and inaccuracies. In addition, collecting and storing facial biometric data raises privacy concerns, as it involves capturing sensitive information about individuals’ unique physical characteristics. Safeguarding this data from unauthorised access, misuse or breaches is critical to maintaining trust and compliance with privacy regulations.
The future of facial biometrics will witness a significant surge in the adoption of deep learning techniques. Deep learning algorithms, fuelled by vast datasets and computational power, will revolutionise facial recognition capabilities, enabling more accurate and robust identification of individuals. By leveraging deep neural networks, facial biometrics systems will achieve unprecedented levels of performance, surpassing traditional methods and enhancing security across various applications. Facial biometrics has the potential to simplify Know Your Customer (KYC) and Customer Due Diligence (CDD) processes through efficient identity verification via ID document scanning and AI facial recognition.
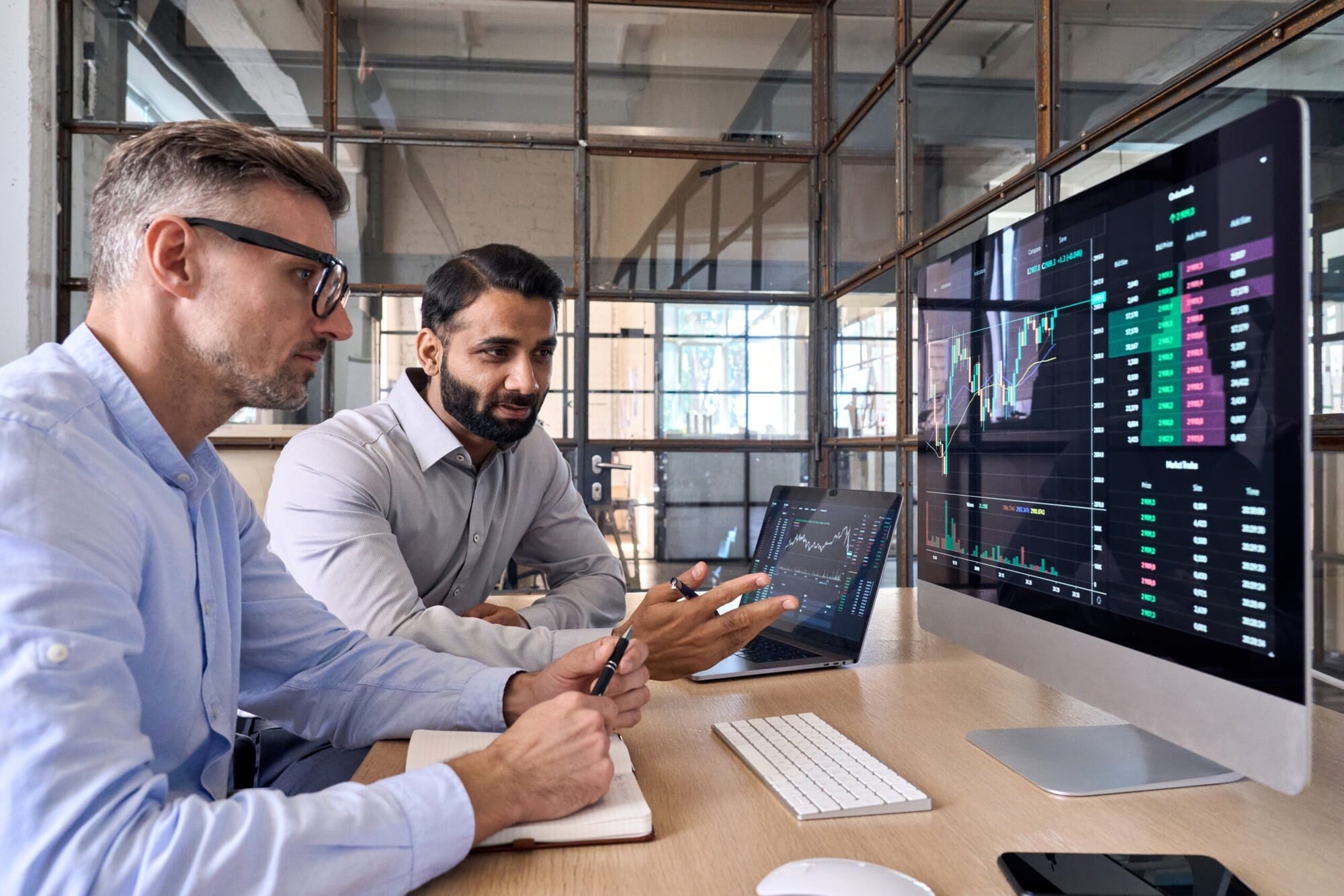
Final Thoughts
Technology will continue to be at the forefront of AML compliance efforts and detection. In particular, artificial intelligence (AI) and blockchain are likely to assume even greater significance moving forward. Leveraging the power of AI to sift through vast volumes of data, detect anomalies and generate real-time insights is providing effective intelligence. However, this vital investment in technology should not be used as a justification for underinvestment in staff and training, as the technology is far from the point where these automated processes are being able to fully replace human interactions.